Statistical Analysis
This notebook contains routines for analyzing the output of keypoint-MoSeq.
Note
The interactive widgets require jupyterlab launched from the keypoint_moseq
environment. They will not work properly in jupyter notebook.
Setup
We assume you have already have keypoint-MoSeq outputs that are organized as follows.
<project_dir>/ ** current working directory
└── <model_name>/ ** model directory
├── results.h5 ** model results
└── grid_movies/ ** [Optional] grid movies folder
Use the code below to enter in your project directory and model name.
import keypoint_moseq as kpms
project_dir='path/to/project' # the full path to the project directory
model_name='model_name' # name of model to analyze (e.g. something like `2023_05_23-15_19_03`)
Assign Groups
The goal of this step is to assign group labels (such as “mutant” or “wildtype”) to each recording. These labels are important later for performing group-wise comparisons.
The code below creates a table called
{project_dir}/index.csv
and launches a widget for editing the table. To use the widget:Click cells in the “group” column and enter new group labels.
Hit
Save group info
when you’re done.
If the widget doesn’t appear, you also edit the table directly in Excel or LibreOffice Calc.
kpms.interactive_group_setting(project_dir, model_name)
Generate dataframes
Generate a pandas dataframe called moseq_df
that contains syllable labels and kinematic information for each frame across all the recording sessions.
moseq_df = kpms.compute_moseq_df(project_dir, model_name, smooth_heading=True)
moseq_df
name | centroid_x | centroid_y | heading | angular_velocity | velocity_px_s | syllable | frame_index | group | onset | |
---|---|---|---|---|---|---|---|---|---|---|
0 | 21_11_8_one_mouse.top.irDLC_resnet50_moseq_exa... | 245.691668 | 210.796020 | -1.217558 | 0.000000 | 0.000000 | 7 | 0 | mutant | True |
1 | 21_11_8_one_mouse.top.irDLC_resnet50_moseq_exa... | 246.797705 | 208.926666 | -1.217558 | -0.079308 | 65.161529 | 7 | 1 | mutant | False |
2 | 21_11_8_one_mouse.top.irDLC_resnet50_moseq_exa... | 246.880092 | 208.750297 | -1.227725 | -0.160751 | 5.839875 | 7 | 2 | mutant | False |
3 | 21_11_8_one_mouse.top.irDLC_resnet50_moseq_exa... | 247.338747 | 206.761270 | -1.240335 | -0.246197 | 61.236711 | 7 | 3 | mutant | False |
4 | 21_11_8_one_mouse.top.irDLC_resnet50_moseq_exa... | 248.073132 | 205.021514 | -1.240335 | -0.336099 | 56.652130 | 7 | 4 | mutant | False |
... | ... | ... | ... | ... | ... | ... | ... | ... | ... | ... |
643906 | 22_27_04_cage4_mouse2_0.top.irDLC_resnet50_mos... | 217.514933 | 196.355583 | 0.193480 | -0.618441 | 565.377392 | 12 | 53618 | default | False |
643907 | 22_27_04_cage4_mouse2_0.top.irDLC_resnet50_mos... | 202.928966 | 183.149695 | 0.086206 | -0.318911 | 590.280706 | 12 | 53619 | default | False |
643908 | 22_27_04_cage4_mouse2_0.top.irDLC_resnet50_mos... | 187.950492 | 169.656667 | 0.193808 | -0.126241 | 604.793226 | 12 | 53620 | default | False |
643909 | 22_27_04_cage4_mouse2_0.top.irDLC_resnet50_mos... | 173.977080 | 155.679578 | 0.302726 | -0.026824 | 592.919672 | 12 | 53621 | default | False |
643910 | 22_27_04_cage4_mouse2_0.top.irDLC_resnet50_mos... | 158.353112 | 143.198606 | 0.136897 | 0.004577 | 599.912268 | 12 | 53622 | default | False |
643911 rows × 10 columns
Next generate a dataframe called stats_df
that contains summary statistics for each syllable in each recording session, such as its usage frequency and its distribution of kinematic parameters.
stats_df = kpms.compute_stats_df(
project_dir,
model_name,
moseq_df,
min_frequency=0.005, # threshold frequency for including a syllable in the dataframe
groupby=['group', 'name'], # column(s) to group the dataframe by
fps=30) # frame rate of the video from which keypoints were inferred
stats_df
group | name | syllable | heading_mean | heading_std | heading_min | heading_max | angular_velocity_mean | angular_velocity_std | angular_velocity_min | angular_velocity_max | velocity_px_s_mean | velocity_px_s_std | velocity_px_s_min | velocity_px_s_max | frequency | duration | |
---|---|---|---|---|---|---|---|---|---|---|---|---|---|---|---|---|---|
0 | default | 21_12_10_def6a_1_1.top.irDLC_resnet50_moseq_ex... | 0 | -0.185726 | 1.675327 | -3.141268 | 3.141216 | 0.006244 | 5.969984 | -188.391070 | 188.423102 | 23.873621 | 18.251097 | 0.052929 | 250.661913 | 0.193452 | 1.278718 |
1 | default | 21_12_10_def6a_1_1.top.irDLC_resnet50_moseq_ex... | 1 | -0.186075 | 1.545966 | -3.138770 | 3.141024 | -0.047082 | 9.326394 | -188.077053 | 3.634033 | 39.028218 | 31.174287 | 0.688662 | 216.440283 | 0.113839 | 0.884532 |
2 | default | 21_12_10_def6a_1_1.top.irDLC_resnet50_moseq_ex... | 2 | -0.270518 | 1.744452 | -3.141173 | 3.140562 | -0.237998 | 12.575613 | -187.851585 | 188.203382 | 61.339926 | 45.208260 | 0.699143 | 249.923269 | 0.130208 | 0.846095 |
3 | default | 21_12_10_def6a_1_1.top.irDLC_resnet50_moseq_ex... | 3 | 0.300797 | 1.392815 | -3.139225 | 3.140805 | -0.005278 | 10.043521 | -188.285953 | 188.290531 | 35.085909 | 27.308359 | 0.488694 | 234.898832 | 0.112351 | 1.552759 |
4 | default | 21_12_10_def6a_1_1.top.irDLC_resnet50_moseq_ex... | 4 | 0.004180 | 1.850509 | -3.136158 | 3.138170 | 0.292483 | 13.734939 | -188.161345 | 188.074944 | 38.752501 | 31.086649 | 0.536752 | 204.338343 | 0.068452 | 1.219203 |
... | ... | ... | ... | ... | ... | ... | ... | ... | ... | ... | ... | ... | ... | ... | ... | ... | ... |
163 | mutant | 22_04_26_cage4_1_1.top.irDLC_resnet50_moseq_ex... | 12 | -0.083118 | 2.002411 | -3.127063 | 3.121900 | 1.286797 | 16.501619 | -11.525765 | 186.041027 | 54.491124 | 35.084645 | 1.652698 | 219.963610 | 0.020379 | 0.280460 |
164 | mutant | 22_04_26_cage4_1_1.top.irDLC_resnet50_moseq_ex... | 13 | -0.460300 | 1.651639 | -2.784471 | 2.309956 | 0.353800 | 1.015509 | -2.823881 | 4.302075 | 41.289938 | 28.827368 | 1.202097 | 199.331569 | 0.011244 | 0.675000 |
165 | mutant | 22_04_26_cage4_1_1.top.irDLC_resnet50_moseq_ex... | 14 | -1.045921 | 2.058440 | -3.125407 | 3.129775 | -0.195097 | 18.098854 | -187.265397 | 184.470467 | 29.156940 | 16.905312 | 0.490859 | 93.623653 | 0.010541 | 0.473333 |
166 | mutant | 22_04_26_cage4_1_1.top.irDLC_resnet50_moseq_ex... | 15 | -0.480634 | 1.747967 | -2.962049 | 2.290607 | -0.912562 | 1.141117 | -2.711569 | 2.690855 | 38.201989 | 19.694319 | 2.358622 | 92.955113 | 0.004216 | 0.588889 |
167 | mutant | 22_04_26_cage4_1_1.top.irDLC_resnet50_moseq_ex... | 16 | -1.007293 | 1.526657 | -3.033172 | 3.117420 | 0.956545 | 14.582857 | -2.738624 | 186.765689 | 39.685048 | 27.693812 | 4.199067 | 161.559462 | 0.004919 | 0.785714 |
168 rows × 17 columns
Optional: Save dataframes to csv
Uncomment the code below to save the dataframes as .csv files
# import os
# # save moseq_df
# save_dir = os.path.join(project_dir, model_name) # directory to save the moseq_df dataframe
# moseq_df.to_csv(os.path.join(save_dir, 'moseq_df.csv'), index=False)
# print('Saved `moseq_df` dataframe to', save_dir)
# # save stats_df
# save_dir = os.path.join(project_dir, model_name)
# stats_df.to_csv(os.path.join(save_dir, 'stats_df'), index=False)
# print('Saved `stats_df` dataframe to', save_dir)
Label syllables
The goal of this step is name each syllable (e.g., “rear up” or “walk slowly”).
The code below creates an empty table at
{project_dir}/{model_name}/syll_info.csv
and launches an interactive widget for editing the table. To use the widget:Select a syllable from the dropdown to display its grid movie.
Enter a name into the
label
column of the table (and optionally a short description too).When you are done, hit
Save syllable info
at the bottom of the table.
If the widget doesn’t appear, you can also edit the file directly in Excel or LibreOffice Calc.
kpms.label_syllables(project_dir, model_name, moseq_df)
Compare between groups
Test for statistically significant differences between groups of recordings. The code below takes a syllable property (e.g. frequency or duration), plots its disribution for each syllable across for each group, and also tests whether the property differs significantly between groups. The results are summarized in a plot that is saved to {project_dir}/{model_name}/analysis_figures
.
There are two options for setting the order of syllables along the x-axis. When order='stat'
, syllables are sorted by the mean value of the statistic. When order='diff'
, syllables are sorted by the magnitude of difference between two groups that are determined by the ctrl_group
and exp_group
keywords. Note ctrl_group
and exp_group
are not related to significance testing.
kpms.plot_syll_stats_with_sem(
stats_df, project_dir, model_name,
plot_sig=True, # whether to mark statistical significance with a star
thresh=0.05, # significance threshold
stat='frequency', # statistic to be plotted (e.g. 'duration' or 'velocity_px_s_mean')
order='stat', # order syllables by overall frequency ("stat") or degree of difference ("diff")
ctrl_group='a', # name of the control group for statistical testing
exp_group='b', # name of the experimental group for statistical testing
figsize=(8, 4), # figure size
groups=stats_df['group'].unique(), # groups to be plotted
);
Saved figure to ../../testing/demo_project/2023_08_01-10_16_25/figures/frequency_stat_stats.png
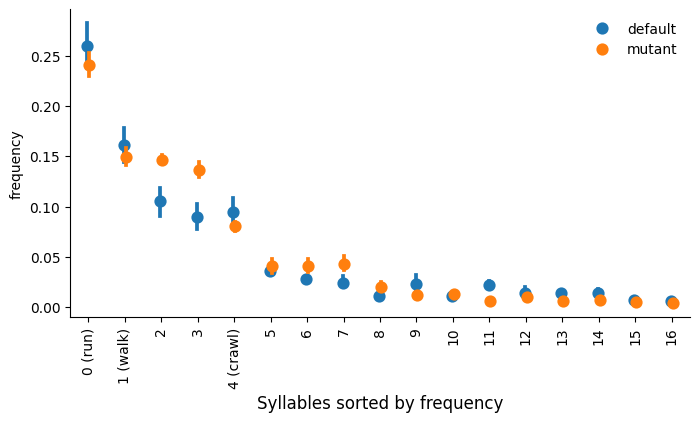
Transition matrices
Generate heatmaps showing the transition frequencies between syllables.
normalize='bigram' # normalization method ("bigram", "rows" or "columns")
trans_mats, usages, groups, syll_include=kpms.generate_transition_matrices(
project_dir, model_name, normalize=normalize,
min_frequency=0.005 # minimum syllable frequency to include
)
kpms.visualize_transition_bigram(
project_dir, model_name, groups, trans_mats, syll_include, normalize=normalize,
show_syllable_names=True # label syllables by index (False) or index and name (True)
)
Group(s): default, mutant
Saved figure to ../../testing/demo_project/2023_08_01-10_16_25/figures/transition_matrices.png
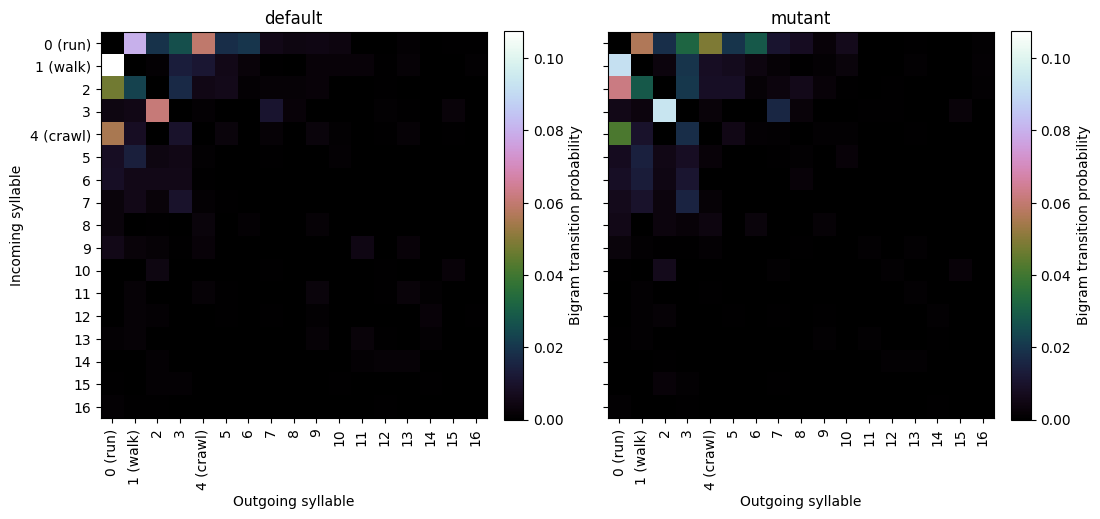
Syllable Transition Graph
Render transition rates in graph form, where nodes represent syllables and edges represent transitions between syllables, with edge width showing transition rate for each pair of syllables (secifically the max of the two transition rates in each direction).
# Generate a transition graph for each single group
kpms.plot_transition_graph_group(
project_dir, model_name,
groups, trans_mats, usages, syll_include,
layout='circular', # transition graph layout ("circular" or "spring")
show_syllable_names=False # label syllables by index (False) or index and name (True)
)
Saved figure to ../../testing/demo_project/2023_08_01-10_16_25/figures/transition_graphs.png
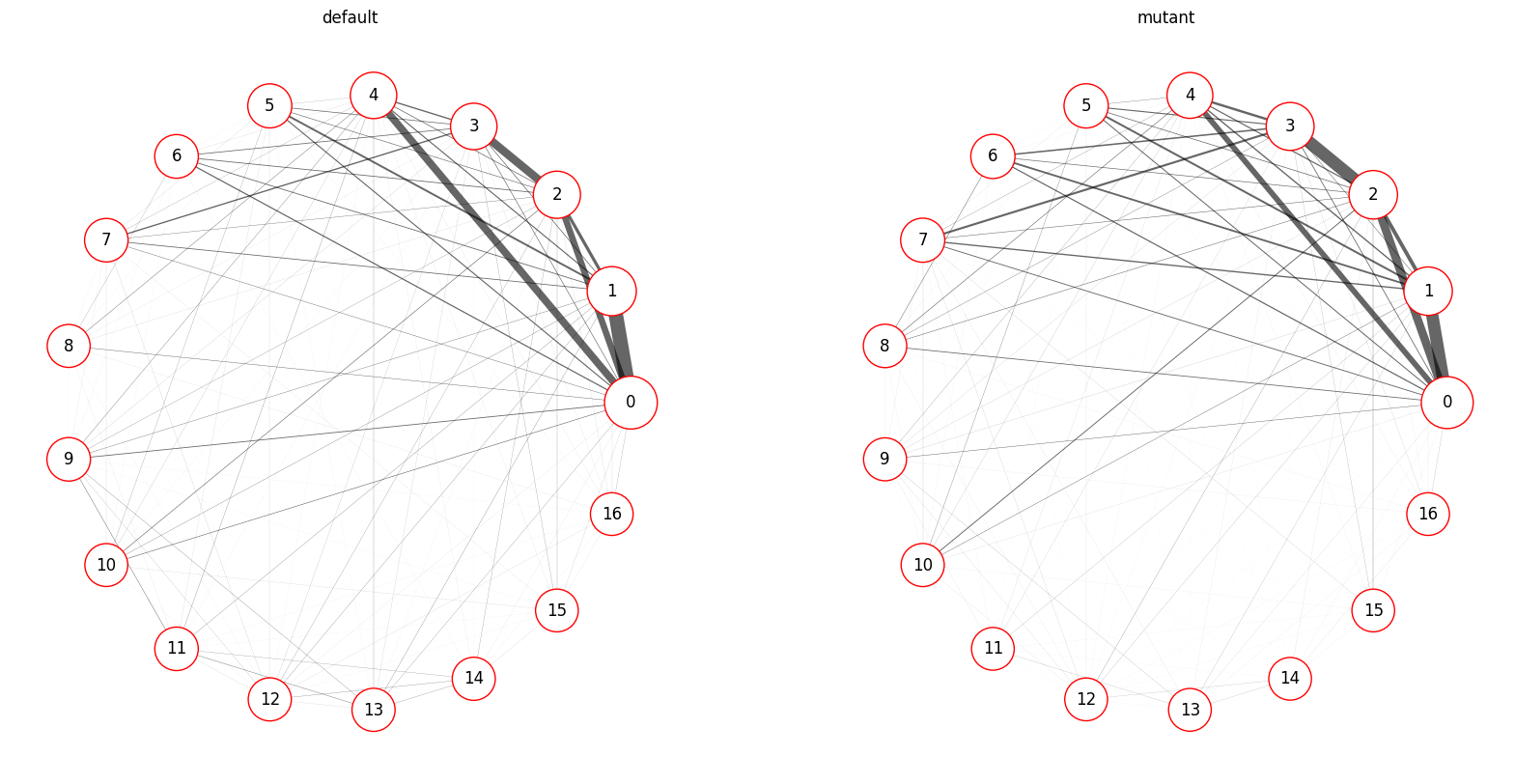
# Generate a difference-graph for each pair of groups.
kpms.plot_transition_graph_difference(project_dir, model_name,
groups, trans_mats, usages, syll_include,
layout='circular') # transition graph layout ("circular" or "spring")
Saved figure to ../../testing/demo_project/2023_08_01-10_16_25/figures/transition_graphs_diff.png
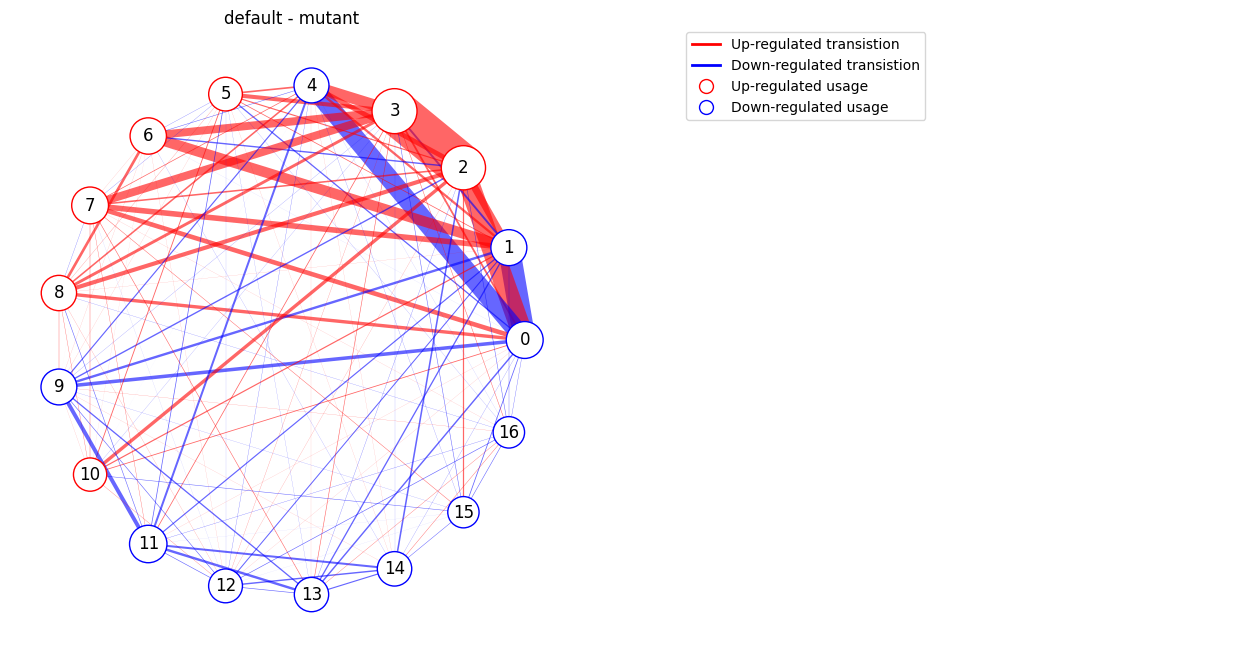